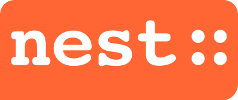
Installing the NEST simulator for use with Anaconda was pretty painless. However, it took me two failed tries to realize just how painless, so I thought I'd post how I got it working.
Note I am in Ubuntu 16/Python 3.7/Conda 4.5.11. If you are on Windows, don't even bother. Just install Linux.
Edit:
Note since I posted this, someone has created an installer that should make installation really easy. I have not tried it, so cannot vouch for it, but I recommend that before you follow my recipe, see the first comment below and give it a shot.
1. Create/activate your nest environment
conda create --name nest
conda activate nest
2. Install system packages you will need
Note this is for the 'standard' configuration as described at
http://www.nest-simulator.org/installation/.
sudo apt-get install -y build-essential cmake \
libltdl7-dev libreadline6-dev libncurses5-dev \
libgsl0-dev openmpi-bin libopenmpi-dev
3. Install python packages
conda install numpy scipy matplotlib ipython nose cython scikit-learn
4. Install NEST proper (see
http://www.nest-simulator.org/installation/)
Obviously you can use whatever directory structure you want, but I put my
build in
/opt/nest, so change your values below accordingly if you want something else.
a. Create folder
/opt/nest and give yourself ownership if needed (I had to use
sudo chown)
b. Download NEST (I put the tarball in /opt/nest)
c. Unpack the tarball (in /opt/nest):
tar -xzvf nest-simulator-2.16.0.tar.gz
d. Create a build directory (again, within opt/nest/):
mkdir nest-simulator-2.16.0-build
e cd to the build directory:
cd nest-simulator-2.16.0-build
f. Run cmake to build makefiles
Note the -Dwith-python=3 option, which forces it to use Python 3.
cmake -DCMAKE_INSTALL_PREFIX:PATH=/opt/nest/ /opt/nest/nest-simulator-2.16.0 -Dwith-python=3
I got some warnings ('Cannot generate a safe linker search path for target sli_readline ') but things seemed to work out ok.
g. Set environment variables
Add the following line to .bashrc:
source /opt/nest/bin/nest_vars.sh
5. Run the makefiles
make #this will take a few minutes(you may get some warnings)
make install #this goes quickly
make installcheck #takes a few miniutes, gives summary at end
6. Test something out
From your favorite IDE or command line, run a simple script (e.g., one_neuron.py in pynest/examples).
Things should just work. I like to use Spyder with conda, so added the following:
conda install spyder
spyder #so spyder runs from the nest environment
The
one_neuron.py example worked just fine. Note, though, if you are using spyder, you will not want to run your code using F5 unless you are a fan of restarting your Python kernel constantly. To avoid problems, I recommend entering
run filename.py in Spyder's (ipython) command line when ready to run a script.
7. Have fun!
Enjoy NEST, it is a really amazing neural simulation framework. I recommend starting here to learn how to program in pynest, the Python interface for the NEST simulator:
http://www.nest-simulator.org/introduction-to-pynest/